.jpg)
In the rapidly evolving realm of AI, we're becoming increasingly aware of its environmental impact during training. According to McKinsey & Company, the tech industry churns out roughly 350 to 400 megatons of CO2-equivalents yearly for enterprises. That’s equivalent to the entire CO2 output of the UK and half of what emanates from aviation or shipping industries. It’s a colossal impact we can’t afford to ignore. Yet, as AI enthusiasts, we all agree that halting AI is not an option. Fortunately, training AI models sustainably is viable, provided conscious decisions are made. By trimming the workload, optimizing server use, and selecting the right timing and location, you can significantly curtail emissions. This approach dovetails with an emerging development vision: GreenOps.
GreenOps stands as a groundbreaking operational management approach emphasizing environmental consciousness and sustainability in the IT sector. It moves beyond just striving for efficiency and performance, aiming to reduce the ecological footprint of server usage. Its core principles involve optimizing energy consumption, cutting waste, and minimizing CO2 emissions. A primary practical implementation is through energy-efficient hardware and fine-tuning server configurations, aligning them precisely with workload needs to prevent excessive energy consumption. GreenOps isn't merely an ethical approach to IT management; it offers tangible benefits to businesses—cost savings, improved operational efficiency, and reduced negative environmental impact—a step towards a more sustainable and responsible IT industry.
In a series of three blogs, we'll guide you through this journey. This inaugural blog presents practical tips to minimize the workload in training your AI models, a significant step in reducing your ecological footprint.
4 Tips for More Efficient AI Model Training Workload
Optimizing your AI model training workload significantly lessens unnecessary environmental strain. Let's delve into concrete steps to optimize your AI training process, reducing your ecological impact:
- Remove Unused or Underutilized Services and Components: Picture a cluttered attic filled with old items—taking up space and serving no purpose. The same applies to unused or underutilized services and components in your AI training infrastructure. They demand continual updates and occupy server space, consuming energy and resulting in CO2 emissions. Removing these surplus elements reduces workload and conserves energy.
- Utilize Required Specifications, Not Maximum Values: Instead of opting blindly for maximum resource requirements, realistically assess what your AI models truly need. It's akin to a thermostat: setting it at maximum when unnecessary wastes energy. Apply the same logic to AI training—choose appropriate resource settings and hardware to prevent excessive energy usage.
- Smart Caching: Caching stores information that might be needed later, reducing repetitive data requests. This principle applies to AI training too. Smart caching minimizes workload and enhances process efficiency by reducing network data, hence lowering energy consumption.
- Build and Write Efficiently by Choosing the Right Language: Selecting the right framework, programming language, and crafting efficient code substantially reduces your workload. However, keep an eye on the entire process, ensuring improvement in one area doesn't deteriorate elsewhere. For instance, compare Golang versus Python or Rust versus Ruby for enhanced efficiency.
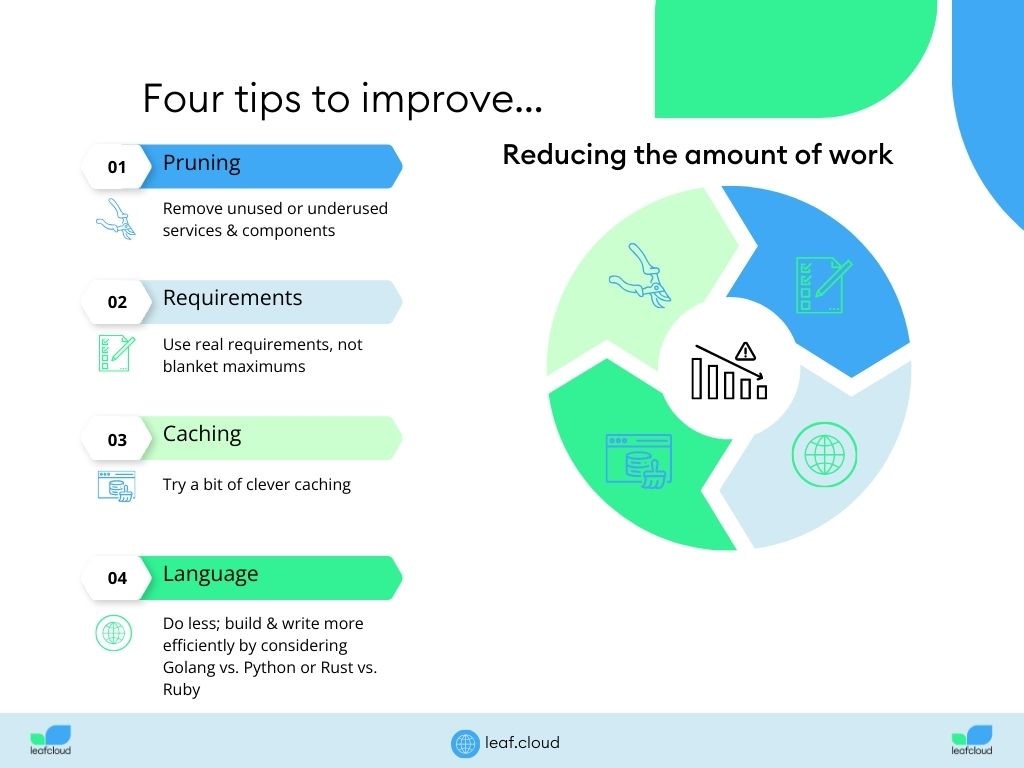
Leafcloud: The Green Revolution in AI Training
At Leafcloud, we strive daily to prevent CO2 emissions instead of merely compensating for them. This endeavor extends to AI model training. Our unique concept involves placing servers in buildings directly linked to water and heating systems, allowing us to reuse almost all residual heat. This brings a double win—reducing fossil fuel usage for building residents while utilizing server energy for both server operations and heating water.
Moreover, by not constructing new data centers, we prevent additional CO2 emissions, marking yet another win-win situation. This approach helps us save up to 1,691 tons of CO2 annually per location—equivalent to the energy consumption of over 200 households. By running your AI model training on Leafcloud servers, you can maintain your AI training and IT usage while being sustainable.
Conclusion: Reduce Workload, Reduce CO2 Emissions
In this blog, we’ve shared tips to lessen the ecological footprint of your AI training. Removing unused elements, realistically determining resource requirements, employing smart caching, and programming more efficiently can train your AI models with minimal environmental impact. In the upcoming blogs, we’ll delve deeper into optimizing server usage and making intelligent choices regarding the timing and location of AI training. Stay tuned for more insights and tips on training AI models with minimal CO2 emissions.
If you genuinely want to train your AI models sustainably and reduce CO2 emissions, opt for Leafcloud as your cloud provider. Together, we can contribute to a greener future for AI training. Read here how the Brunel Solar Team uses our cloud to calculate CO2-negative assessments for their solar car. Feel free to reach out via email or phone to discuss what works best for you, obligation-free.